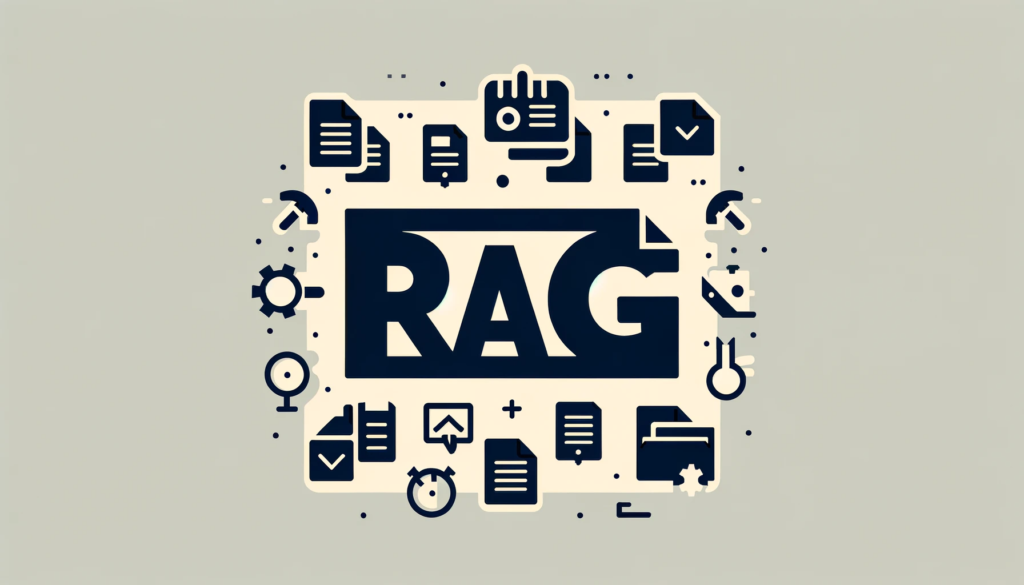
Introduction
In the evolving landscape of artificial intelligence (AI) and machine learning, Retrieval-Augmented Generation (RAG) has emerged as a groundbreaking technology. RAG combines the strengths of retrieval-based systems and generative models, revolutionizing how AI generates responses by referencing authoritative knowledge bases. This article delves into RAG’s intricacies, its operational mechanisms, and its pivotal role in synthetic research, enhancing data accuracy, relevance, and overall research efficiency.
Understanding Retrieval-Augmented Generation (RAG)
Definition of RAG
Retrieval-Augmented Generation (RAG) optimizes the output of large language models (LLMs) by referencing authoritative external knowledge bases before generating responses. Unlike traditional LLMs, which rely solely on their training data, RAG integrates real-time data retrieval to produce contextually rich and accurate outputs, making it a cost-effective solution for improving LLM performance across various domains.
How RAG Works
RAG operates through a two-step process: retrieval and generation. Initially, the model retrieves relevant information from external data sources using advanced search algorithms. This data is then processed by the generative model to formulate comprehensive responses. This dual mechanism ensures that the generated content is both accurate and enriched with current, relevant information.
The Mechanics Behind RAG
Integration of Retrieval and Generation
The core functionality of RAG lies in seamlessly blending retrieval and generation. During the retrieval phase, the model accesses a database or the internet to fetch pertinent information. The generative model then synthesizes this data into a coherent response, leveraging the interplay between these phases to create responses that are informed and contextually appropriate.
Advantages Over Traditional Models
Traditional AI models often struggle with generating responses that are both accurate and current. RAG addresses these limitations by incorporating real-time data retrieval, significantly enhancing the relevance and accuracy of its outputs. This capability is particularly beneficial in fields where timely and precise information is critical, such as synthetic research.
Importance of RAG in Synthetic Research
Enhancing Data Accuracy
In synthetic research, data accuracy is paramount. RAG’s ability to retrieve and integrate the latest information ensures that researchers have access to the most current data available, reducing the risk of outdated or inaccurate information and enhancing the overall quality of the research.
Improving Research Efficiency
RAG’s dual functionality significantly improves research efficiency by automating the retrieval and synthesis of information. This allows researchers to focus more on analysis and interpretation rather than data collection, a crucial aspect in synthetic research where timely and accurate data collection is essential for successful outcomes.
Facilitating Complex Data Synthesis
Synthetic research often involves synthesizing complex and diverse data sets. RAG excels in this domain by retrieving relevant data from various sources and integrating it into unified responses. This capability enables researchers to tackle complex research questions more effectively, leveraging a broad spectrum of information to derive meaningful insights.
Applications of RAG in Synthetic Research
Drug Discovery
In drug discovery, the ability to quickly access and synthesize relevant information is critical. RAG can retrieve the latest research findings, clinical trial data, and biochemical information, aiding researchers in identifying potential drug candidates more efficiently.
Material Science
Material science research often requires integrating diverse data sets, ranging from experimental results to theoretical models. RAG facilitates this integration, providing researchers with comprehensive insights that inform the development of new materials and technologies.
Market Research
Market research stands to benefit immensely from RAG technology. Accurate, up-to-date information is essential for making informed business decisions, and RAG’s ability to pull in the latest market trends, consumer behavior analytics, and competitor data ensures that businesses remain competitive. Let’s delve into the specific benefits of RAG in market research.
Enhancing Market Research Accuracy
Real-Time Data Retrieval
Market conditions are constantly evolving, and decisions based on outdated information can lead to significant setbacks. RAG allows market researchers to retrieve the latest data from various sources such as market reports, social media analytics, and news articles, ensuring that the information used for analysis is current and relevant.
Comprehensive Data Synthesis
Market research often requires synthesizing data from multiple sources, including sales data, consumer feedback, and economic indicators. RAG can integrate this diverse data into coherent insights, providing a more comprehensive view of the market landscape.
Improving Market Research Efficiency
Automated Data Collection
Traditional market research methods often involve time-consuming data collection processes. RAG automates this process, enabling researchers to gather vast amounts of data quickly and efficiently. This allows for more timely analysis and quicker decision-making.
Enhanced Analytical Capabilities
By integrating real-time data retrieval with generative models, RAG enhances the analytical capabilities of market researchers. It enables them to perform more complex analyses, such as sentiment analysis or trend forecasting, which are crucial for strategic planning and competitive analysis.
Facilitating In-Depth Market Insights
Consumer Behavior Analysis
Understanding consumer behavior is key to successful marketing strategies. RAG can retrieve and analyze data from social media, online reviews, and purchase histories, providing deep insights into consumer preferences, purchasing patterns, and emerging trends.
Competitor Analysis
Staying ahead of competitors requires continuous monitoring of their activities. RAG can retrieve the latest information on competitor products, marketing strategies, and financial performance, helping businesses to anticipate market movements and adjust their strategies accordingly.
Challenges and Limitations of RAG
Data Quality and Reliability
One of the primary challenges associated with RAG is ensuring the quality and reliability of the retrieved data. Since the model relies on external sources, the accuracy of its outputs is contingent upon the trustworthiness of these sources. Implementing robust data validation mechanisms is essential to address this challenge.
Computational Complexity
The integration of retrieval and generation phases introduces additional computational complexity, leading to increased processing times and resource requirements. Optimizing these processes is crucial to maintaining the efficiency and practicality of RAG in research applications.
Ethical Considerations
The use of RAG also raises ethical considerations, particularly concerning data privacy and security. Ensuring that the retrieved data is used responsibly and in compliance with ethical standards is crucial to maintaining the integrity of synthetic research.
Future Prospects of RAG in Synthetic Research
Advancements in Algorithm Design
Ongoing advancements in algorithm design are expected to enhance the capabilities of RAG. Improved retrieval algorithms and more sophisticated generative models will enable the creation of even more accurate and contextually rich responses, further benefiting synthetic research.
Integration with Other Technologies
The integration of RAG with other emerging technologies, such as quantum computing and blockchain, holds significant promise. These integrations could enhance the efficiency, security, and accuracy of RAG, opening new avenues for its application in synthetic research.
Expanding Research Domains
As RAG technology continues to evolve, its application is likely to expand into new research domains. Fields such as environmental science, social sciences, and humanities could all benefit from the enhanced data retrieval and synthesis capabilities offered by RAG.
Conclusion
Retrieval-Augmented Generation (RAG) represents a significant leap forward in the field of artificial intelligence, offering a powerful tool for synthetic research. By combining the strengths of retrieval-based systems and generative models, RAG enhances data accuracy, improves research efficiency, and facilitates the synthesis of complex data sets. Despite its challenges, the potential of RAG to revolutionize synthetic research is immense, promising exciting advancements and new possibilities for the future.
A notable example of RAG’s practical application is Personno.ai, a market research platform leveraging AI respondents. By integrating RAG technology, Personno.ai provides businesses with real-time, accurate market insights and consumer behavior analytics, enhancing decision-making processes and ensuring competitive advantage.
FAQs
What is Retrieval-Augmented Generation (RAG)?
RAG is a hybrid AI model that integrates retrieval and generation techniques to produce contextually rich and accurate responses by accessing external data sources.
How does RAG improve synthetic research?
RAG enhances synthetic research by providing up-to-date information, improving data accuracy, and streamlining the data synthesis process, thereby increasing research efficiency.
What are the main challenges of using RAG?
The primary challenges include ensuring data quality and reliability, managing computational complexity, and addressing ethical considerations related to data privacy and security.
In which research fields can RAG be applied?
RAG can be applied in various fields, including drug discovery, material science, market research, environmental science, social sciences, and humanities.
How does RAG differ from traditional AI models?
Unlike traditional AI models that rely solely on pre-existing datasets, RAG incorporates real-time data retrieval, enhancing the relevance and accuracy of its outputs.
What are the future prospects of RAG in synthetic research?
Future prospects include advancements in algorithm design, integration with other emerging technologies, and the expansion of RAG’s application to new research domains.